Efforts to track battery value through raw materials and simplify battery data identification have been challenging — but significant progress is being made. Read the article to learn more about Axel's projects, how they took shape, and how they align with Cling's mission to establish the platform for battery circularity at scale.
1. Introduction
2. Project 1: Raw Material Estimator
3. Project 2: AI Vision Model
4. Contributions to Cling's Mission
5. Conclusion
Introduction
Axel Berglund, aka Ax, Data Scientist at Cling, works while pursuing a Master’s degree in Machine Learning at Kungliga Tekniska högskolan. His journey at Cling began with a strong focus on AI and machine learning. However, through practical experiences and many inspiring conversations with his supervisor, Rasmus Lindqvist, he discovered a new enthusiasm for Data Engineering. This article breaks down the key projects Ax has been working on during the last year at Cling, showcasing his contributions to advancing the company's mission of sustainability and innovation within the EV battery industry.
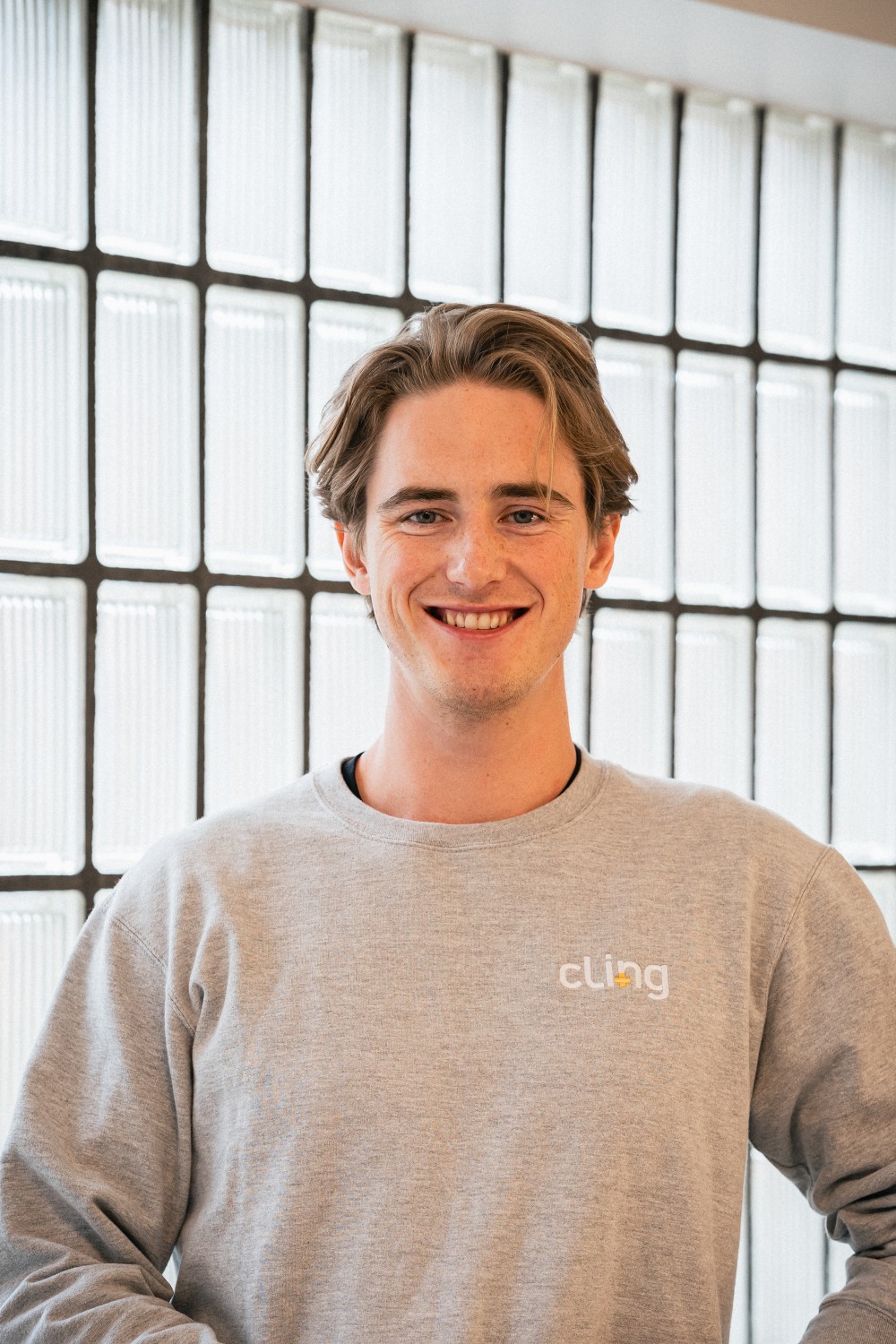
Project 1: Raw Material Estimator
Addressing the Need for Raw Material Tracking in EV Batteries
The residual value of EV batteries is significantly impacted by their material content, which varies greatly among different batteries. This variation is influenced by factors such as cell chemistry, type, size, and voltage capacity. Accurate estimation of raw material content is crucial for valuing battery owners' assets and optimizing sourcing for recyclers. Recyclers often set their processes according to precise chemical compositions, making it vital to know the exact content of batteries.
Ax explains, “Currently, there appears to be a lack of accessible databases with reliable battery data. Even with the upcoming event of battery passports, there will still be a valuable role for passport aggregators who can create additional services using these passports. A material estimator is an excellent starting point for developing such services. By linking material values to the London Metal Exchange (LME), this tool can provide direct access to value estimations, enhancing its practicality and value.”
Building the Model
Cling Systems has built a comprehensive database containing extensive battery data. However, the challenge of obtaining the raw material composition data for each battery remains. To address this, Ax developed an ensemble of models capable of estimating the commodity quantities in different batteries based on extensive research on both pack and cell levels.
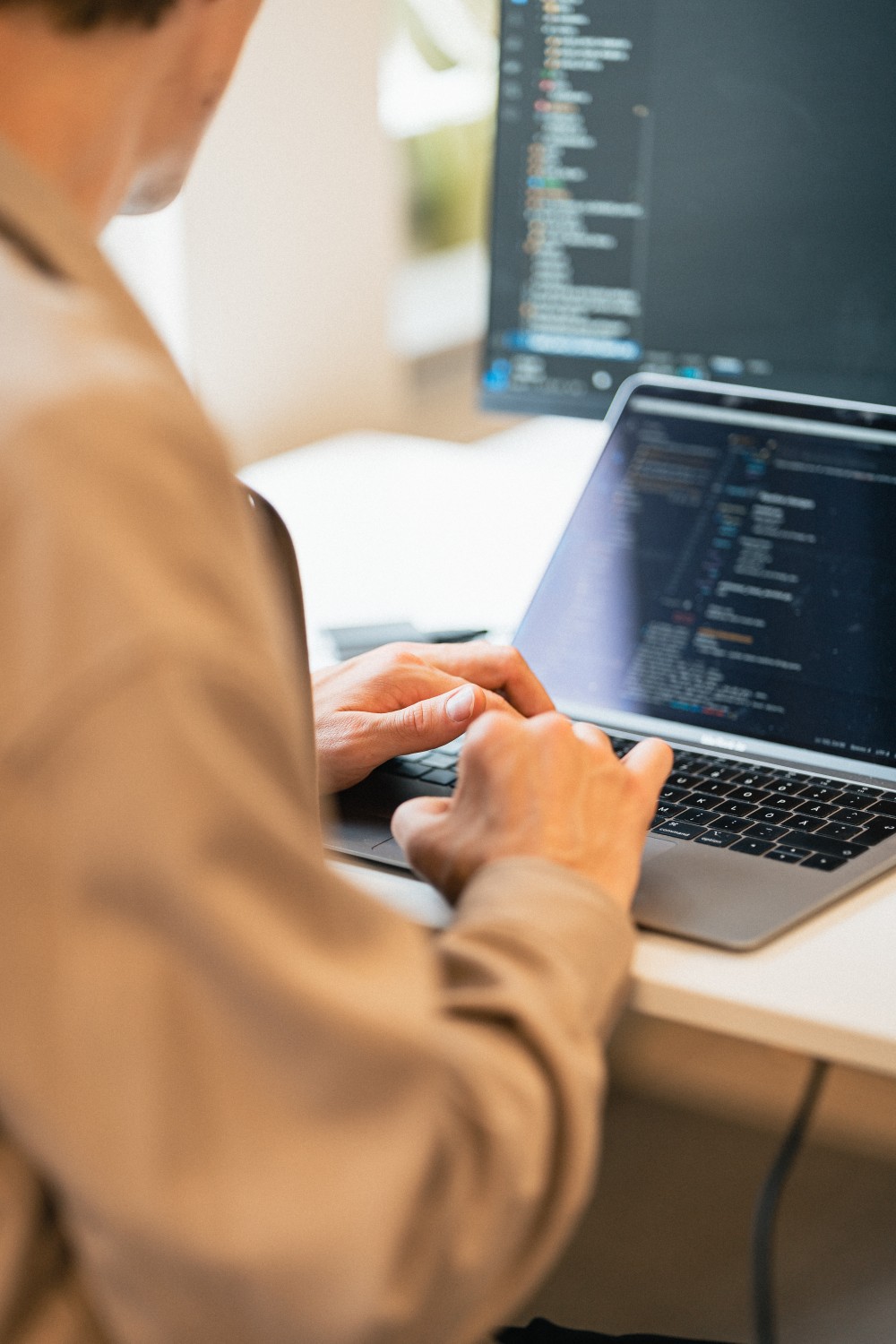
Training the Model
The training data was sourced from extensive research of previous works available online. Despite limited resources and the absence of a strict baseline, Ax successfully built multiple models for the majority of the batteries Cling works with. These models can perform estimations on both pack and cell levels, leveraging features such as battery type, weight, chemistry, and performance characteristics to predict the material contents of lithium, cobalt, nickel, manganese, copper, and aluminum.
Future Work
Future work includes continuously updating the models with new data, employing more advanced machine learning techniques and ensemble learning approaches, and further validating the models to enhance their accuracy.
Challenges and Future Potential
The primary challenge faced was the sparse data available in this relatively new market. However, the potential for this project is immense:
- Infrastructure for Recyclers: Building robust infrastructure will facilitate transparency and efficiency in the recycling process.
- Transparency: More data will allow for more accurate models, enabling stakeholders to know exactly what a battery is made of, which is beneficial for both buying and selling batteries.
- Innovation: As more data becomes available, the models will continue to improve, contributing to circular progress by making recycling processes more efficient and cost-effective.
Project 2: AI Vision Model
Simplifying and Enhancing the Battery Identification Process
Given the rapid growth and diversity of batteries in the market, uploading detailed information about batteries to the Cling platform can be a daunting task for users. To address this, Ax developed an AI vision model to simplify the uploading process by automatically identifying batteries from images. However, the model's capabilities extend far beyond mere uploading; it plays a crucial role in organizing and managing image data, essential for maintaining the quality and transparency of the database.
The goal was to leverage extensive image data of batteries to develop an AI vision model capable of identifying different types of batteries, streamlining the uploading process on the Cling platform, and enhancing overall data management.

Development and Achievements
To develop this model, Ax utilized Vertex AI, a machine learning platform by Google Cloud. Vertex AI provides the tools necessary for building, training, and deploying machine learning models efficiently, allowing for the processing of large datasets of battery images and the training of a highly accurate vision model.
“We now have a trained model capable of identifying 42 different types of batteries, which are the most frequently used on Cling's platform,” says Ax. “This model simplifies the process for users, who can upload their batteries by simply taking a picture of them. Additionally, one of the models was developed specifically for image classification, allowing us to filter out bad images and categorize them efficiently. This capability is crucial as our database grows and handling images becomes increasingly complex.”
Challenges
The primary challenges included image data preprocessing and cleaning, essential steps to ensure the model's accuracy and reliability.
Future Vision and Potential
The potential of the AI vision model extends beyond user-friendliness:
- User Experience: Simplifying the battery identification process enhances user experience, making the platform more accessible and efficient.
- Image Data Management: The model's ability to classify and filter images ensures high-quality data in Cling’s database, streamlining the management process as the database scales.
- Transparency: Automated labeling and quality checks of images improve transparency regarding the state of the batteries, which is essential for end-of-life trade and building trust among stakeholders.
- Infrastructure for Pretrained Models: Developing a robust infrastructure for pretrained AI vision models can significantly benefit the EV battery recycling industry by automating and streamlining various processes.
Contributions to Cling's mission
Ax’s work directly contributes to the company's mision by enhancing the efficiency and effectiveness of recycling processes. He explains, “The Raw Material Estimator and AI Vision Model support Cling's broader mission by improving transparency and reducing costs, and promoting a circular economy. These models help in making informed decisions about recycling, repurposing, and reusing batteries, ultimately reducing environmental impact.”

Future Directions
Potential future directions include:
- Enhancing the accuracy of the Raw Material Estimator with more data and advanced algorithms.
- Expanding the AI Vision Model to identify a wider range of battery types and conditions.
- Developing new models to address other challenges in the EV battery recycling industry.
“My goal is to contribute to Cling's mission of driving sustainability and innovation in the EV battery recycling industry, ensuring that valuable raw materials are efficiently recovered and reused, enabling circularity at scale,” says Ax.
Conclusion
At Cling, the purpose is to drive sustainability and innovation in the EV battery recycling industry. Through projects like the Raw Material Estimator and the AI Vision Model, Ax has contributed to leveraging data, machine learning, and AI to address the complex challenges of recycling EV batteries. Cling’s comprehensive approach to trading, tracing, and transporting, ensuring that valuable raw materials are efficiently recovered and reused, reducing environmental impact and promoting sustainability.
"As we look to the future, my focus will remain on enhancing our models with more data, improving transparency, and developing innovative solutions that drive the industry forward. The journey is challenging, but the potential rewards for sustainability and innovation are immense."
More information
Register to Cling’s platform here.
Interested in learning more about these projects or Cling's services? Get in touch here.